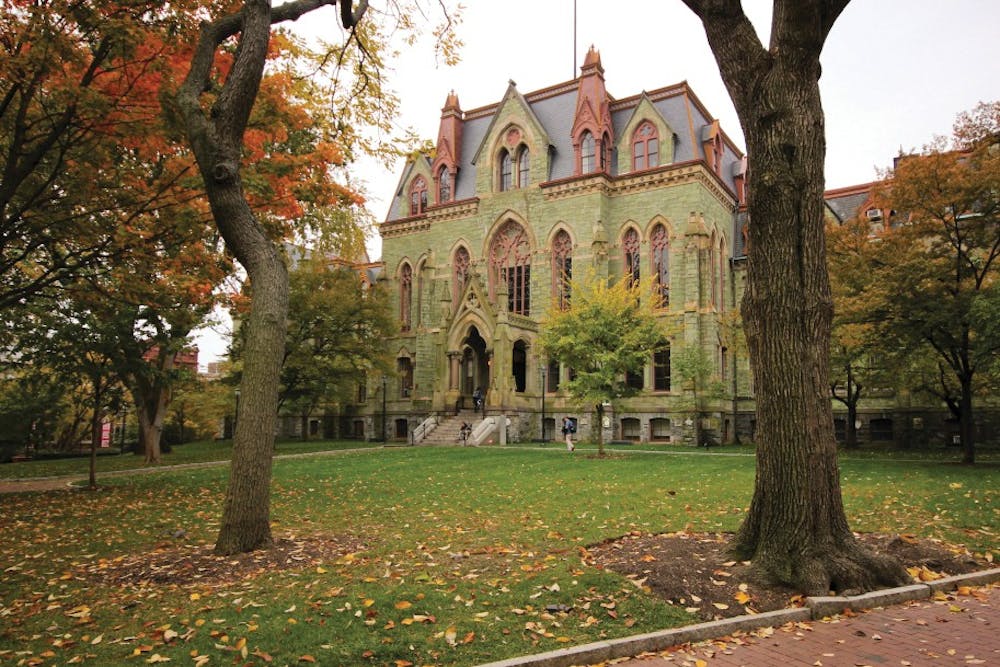
Sorenson and Berk analyzed domestic violence arraignments and found that machine-learning forecasts better identify patterns of human behavior. | DP File Photo
Two Penn professors found that the use of machine-learning forecasts at domestic violence arraignments could substantially reduce repeat domestic violence arrests.
Social policy professor Susan B. Sorenson of social policy and criminology and statistics professor Richard Berk looked at 28,646 domestic violence arraignments that led to charges and releases between January 2007 and October 2011. The researchers conducted a two-year follow-up for each case.
Normally, in the United States, after being arrested, suspects are brought before a judge or magistrate in an arraignment, during which the suspect receives a written document with the list of charges. At this arraignment, the judge or magistrate decides whether the suspect can be released to society.
This decision, pursuant to the Bail Reform Act of 1984, is based on the suspect’s risk of flight and the threat posed to public safety.
The study noted that current decisions are based on limited information and inferences, ignoring information about the offender’s behavior. Machine-based learning processes can combine and understand different inputs that may seem irrelevant to humans.
“Machine-learning is a ‘black-box’ statistical technique that basically squeezes all of the information out of data and is able to identify patterns across many more variables than we humans can understand,” Sorenson said.
“There are many parts of the criminal justice system that are using very recently-developed tools, including machine-learning, to predict who is a ‘good risk,’” Sorenson said. “The arraignment process, particularly for domestic violence [cases], has not had that benefit.”
Their study defines domestic violence as an “intimate relationship including dating, or a familial or blood relationship.” Domestic violence is thus not limited to solely intimate partners.
They looked at inputs such as age, gender, prior charges, age at first charge, prior failures to appear and number of instant drug crime counts, among many other inputs.
Sorenson and Berk sought to find a group of offenders who could be released without concern that they would be rearrested for domestic violence.
“Even a few days in jail when incapacitation is not needed can cost an offender his or her job and more generally disrupt a variety of important household functions (e.g., childcare),” the study said.
Sorenson and Berk also found that less than 19 percent of domestic violence offenders are rearrested for new charges during the two-year follow-up period.
According to the study, “about 40 percent of all individuals arraigned for domestic violence can be released with the expectation that only about 1 in 10 will be rearrested for domestic violence.”
The use of machine-learning could reduce the amount of people who are detained without posing a threat to society.
“We don’t want to have people in jail who don’t need to be in jail,” Sorenson said. “It’s hard for them, it’s hard for their families, and it’s also hard for the taxpayers.”
“Another benefit is that you are able to better identify those who are likely to reoffend,” Sorenson added. In domestic violence, “it’s usually a specific suspect ... who assaults another specific individual.”
If the courts employ this machine-based learning with domestic violence cases, they can decrease the risk posed to those specific individuals.
Sorenson also explained that domestic violence cases differed from other crimes. Unlike robbers who can attack different locations, domestic violence offenders often assault the same person. If the court can predict which offenders are likely to assault that same person again, the court can protect the victim.
“What comes next is [that] the court system needs to decide how they’re going to integrate this into their decision-making,” Sorenson said.
The Daily Pennsylvanian is an independent, student-run newspaper. Please consider making a donation to support the coverage that shapes the University. Your generosity ensures a future of strong journalism at Penn.
DonatePlease note All comments are eligible for publication in The Daily Pennsylvanian.